A year ago, we set out to help B2B go-to-market teams make better decisions by building the best multi-touch attribution model in the world. We failed. But that turned out to be a good thing.
The reasons why we failed were both surprising and illuminating. Across all of our conversations, for every industry and persona, we keep hearing the same thing: it doesn’t matter how advanced a team’s attribution solution is today — no one is ever HAPPY with it. Attribution is simply a necessary evil in order to keep the trains running on time.
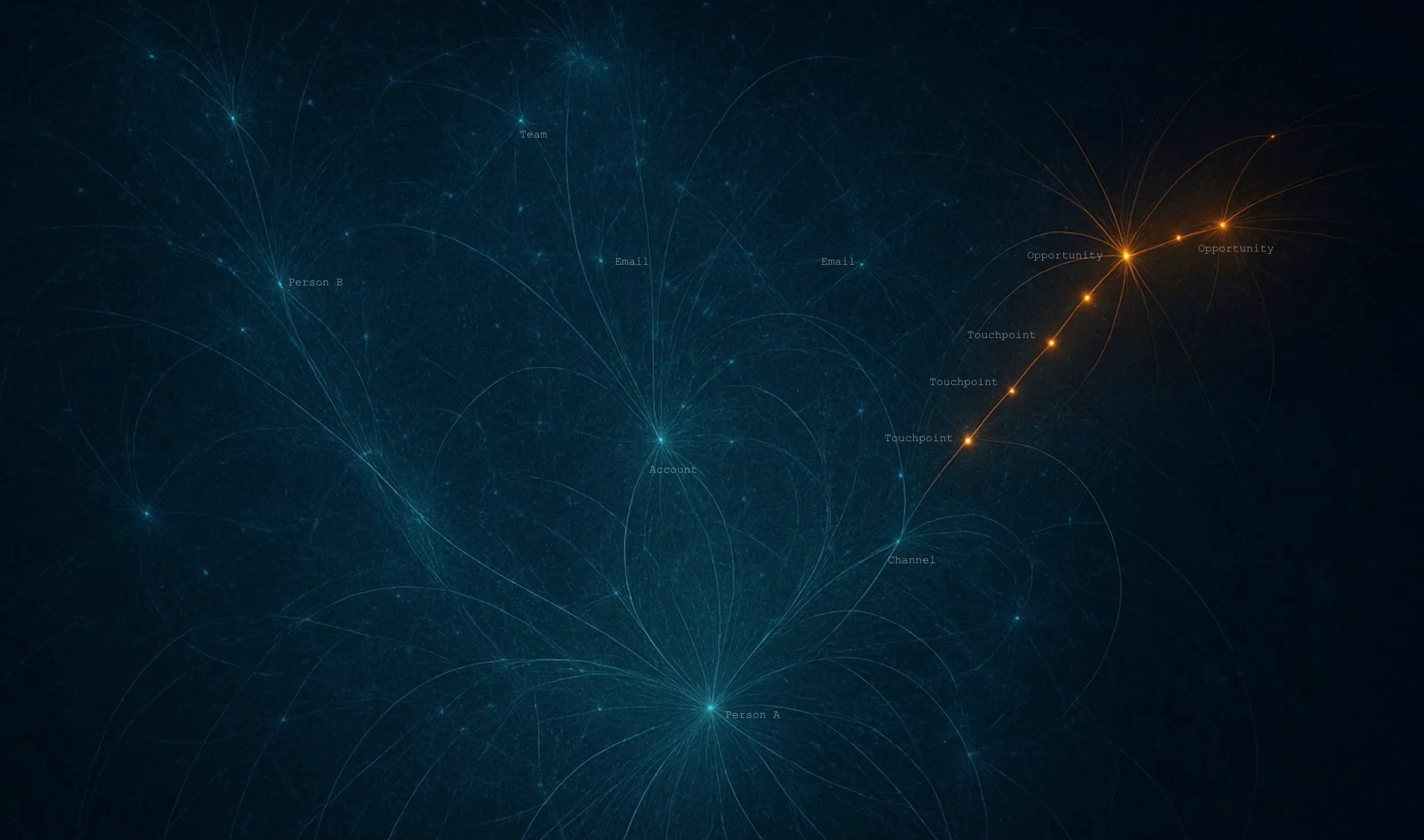
Even Upside’s best-in-the-world multi-touch model became just the least-bad option, and we noticed it still wasn’t enough to help go-to-market teams with their most important questions:
- “What really happened in our deals?”
- “What worked?”
- “How much did it cost us to acquire this customer?”
- “Where should we spend an extra $100k next quarter?”
- “What is not performing, and what can I cut to make space for new initiatives?”
- “How can we increase our pipeline without a bigger budget?”
- “Which half of our GTM spend is actually driving results?”
These are the questions our customers need to answer for their board meetings, and to close more lucrative deals, but these aren’t the insights that come out of any attribution model that exists today.
You can’t answer “what worked” if you don’t know what happened.
After working with our design partners for the past year, we know what’s missing: you can’t answer “what worked” if you don’t know what happened. Relying on better sales rep hygiene, or even more rigorous list import guidelines for marketing managers cannot be the solution. But we’ve discovered that you CAN find game-changing insights that help you make decisions…if you find a way to consider the full story of what happened, as experienced by your customers.
The future of attribution doesn’t look like its past
For the past six months, we’ve been researching two ground-breaking solutions to the problem of B2B measurement:
- A radical improvement to data recovery and healing. Next-generation attribution must be built on next-generation data, because what’s captured in Salesforce or HubSpot simply isn’t good enough for this level of quantitative analysis. And this isn’t something you can do in a spreadsheet or a Looker dashboard either — getting it right involves using the latest in AI and graph theory to extract all the buried signals in your raw data.
- Deal story analysis powered by AI agents. With careful training, a team of AI agents can replicate the investigative rigor of expert data analysts to unlock insights from your data — even when it is poorly-structured chaos that can’t support quantitative analysis — and make astoundingly perceptive qualitative assessments about the nuanced drivers behind conversions. This isn’t just uploading a Salesforce export into ChatGPT and asking questions, because you need the AI to follow the same forensic process that an experienced data analyst would use.
Neither of these approaches would have been technically feasible even a year ago, but state-of-the-art is moving quickly and we’ve already seen outstanding results from both. In this article, we will break down how they work, and why we believe this is the future of B2B revenue attribution and intelligence.
And whether you’ve been struggling to solve your company’s revenue measurement challenge for years, or you have a solution that is already working well, or even if attribution is just something you have strong opinions about, we are passionate about this topic and we would love to hear from you. Please drop us a note!
Why attribution fails today: messy data, subjective weightings, and “accurately” measuring the wrong things
The attribution model we spent most of last year testing was based on an in-house tool we created while at Branch, which had helped us scale that company to over $100 million in revenue (and a valuation of $4 billion), primarily through complex enterprise deals with long cycles and large buying committees.
It was an impact-weighted attribution model, and it worked as designed — that wasn’t the issue. Viewed through the frame of traditional B2B attribution, our results were more accurate and more sensitive than anything else out there. But if you spend time trying to build a multi-touch attribution model, you’ll eventually run into at least one of these brick walls:
Problem 1: Messy data is kryptonite to trustworthy insights
B2B enterprise data is always a disaster. Attribution is a garbage-in-garbage-out problem, and teams seem almost perversely proud to tell us how screwed up their data is!
- Sales reps and marketing managers log and structure touchpoints in ways that help their workflows, not the way customers actually interact with the company (for example, adding people to campaigns days after an event happened).
- People are busy with their day jobs, so even benign requests, such as for AEs to add important people to their deals as contact roles, becomes a distracting and distasteful chore.
- Email is the lowest common denominator of any B2B go-to-market motion, which means it contains a goldmine of useful signals. But email tracking is a cemetery of ambition — tools like Gong still struggle to dedupe the same customer reply in three different AE inboxes, or extract quoted email messages buried deep in a forwarded email chain.
- Every time team leadership changes, or the company upgrades to a more capable marketing automation platform, or someone is tasked to “clean up the campaign list,” data goes missing and classification guidelines get updated. The result is campaigns logged multiple times in different systems, channel values changing every year, and the same prospect appearing as multiple contacts with different email addresses.
Here’s an example of how easily this messy data problem can lead you astray:
What your systems captured:
- Sean M, a prospect, registered for an event but didn’t show up.
- The AE sent an email to him and his CEO.
- There was an inbound email 15 days later from a new contact on this account.

To an attribution model, this collection of touchpoints looks like a direct inbound contact and a deal with only the sales team involved.
What actually happened:
- Sean M and Edith H were invited to a Warriors Game.
- Sean M registered for the Warriors game.
- Edith H attended the warriors Game.
- Edith H had a conversation about CompanyA with Rob P, the COO.
- Dylan followed up with Edith H.
- Edith forwarded the email to Jennifer D, the right person at Company A.
- Jennifer D sent an email to Dylan expressing interest.

In reality, this deal was probably sparked by a VIP event that included an executive assist from the COO, with outbound follow-up help from the AE. All of the relevant details exist somewhere in the deal story, they’re just buried and not legible to any attribution model we’ve ever seen. This muddling of deal drivers is a problem for every go-to-market team, but the impact is particularly evident in marketing — marketers rarely get the credit they deserve in bringing deals, and the CMO role is the shortest-tenure C-level role out there.
Marketers rarely get the credit they deserve in bringing deals, and the CMO role is the shortest-tenure C-level role out there.
Problem 2: There is no objective “right answer” to multi-touch attribution weightings
Our typical customer has somewhere between 2,000 and 10,000 touchpoints per account. Some of these are clearly more important than others, but it’s nearly impossible — or it at least takes many hours — to look at all of them and untangle what helped close the deal and what was just noise along the way. Is an email from sales worth the same if it happens before deal qualification vs. during the negotiation phase? What about a thought-leadership webinar vs. product content? Should these activities always get the same weighting on every deal, or does it depend?
No human could write enough rules to cover all of these scenarios, and there is a significant risk of the “infinite shoreline problem” where more touchpoints are created simply by spending more time to define more rules. But more statistically-rigorous approaches, such as a regression analysis, quickly run out of steam if you can’t feed them clean data about what happened.
Problem 3: Attribution makes it too easy to focus on the wrong questions
When looking for credit and ways to quantify a team’s performance, the concept of “I was first!” is easy to understand. Everybody from an SDR to the CEO gets how that model works. It’s also obviously incomplete, which leads to plenty of “common-sense suggestions” from everyone involved about how to make things better. This is often what leads to an extended session in the B2B attribution hamster wheel, and no one is ever happy at the end.
Fortunately, we’re now in the age of efficient growth, which is inspiring go-to-market leaders to think differently. As one CMO put it to us recently:
“I feel like attribution — the way we’ve been talking about it for years — is kind of old-fashioned. Remember how obsessed we used to be with marketing-sourced and SDR-sourced? People just aren’t thinking that way anymore. Now it’s all about pipeline, and how do I understand what’s working and what’s not so I can make better decisions.”
AI can’t work miracles, but an infinite supply of “interns” sure helps
Last fall, for one of our design partners, we compiled an audit of their most common opportunity activation reasons. We’re highly-experienced go-to-market leaders with data analysis skills, but this project took us weeks and required laboriously poring through thousands of touchpoints by hand.
Don’t expect to upload an unstructured dataset into ChatGPT and get good results
Today, our team of AI analysts can run an equivalent “milestone analysis” in about five minutes, and it regularly finds buried influences even expert human analysts might miss. This is very exciting, because we see deal stories as another form of attribution, even if not expressed in dollars and cents. An analyst-generated story essentially provides a shortcut to answering some of our customers’ most important questions without getting entirely stymied by the messy data problem.
Our preferred format generates an opinion about what led up to the milestone, and then supports that opinion with specific citations from the deal story to validate that the AI team hasn’t manufactured evidence out of thin air:
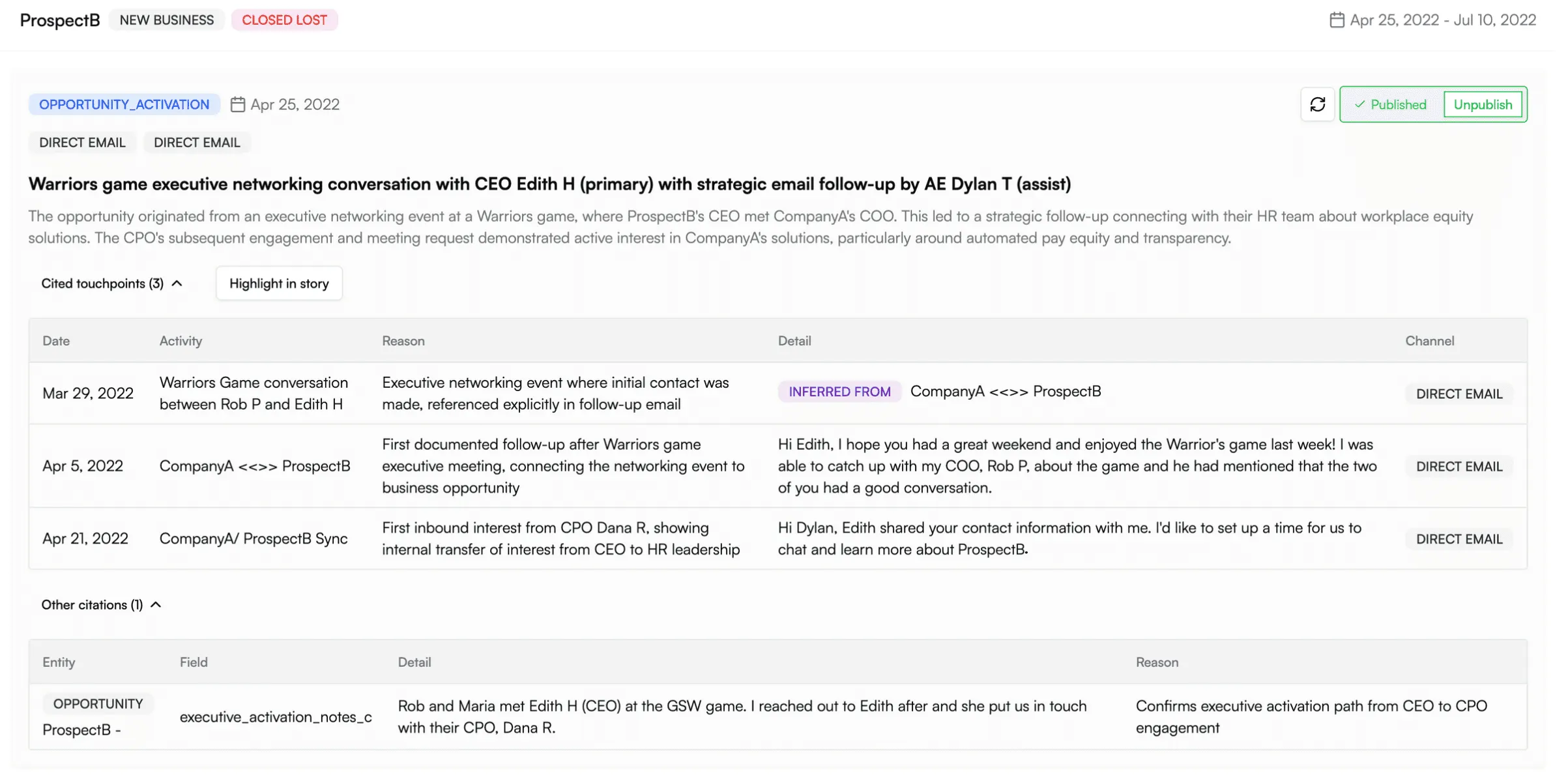
However, don’t expect to upload an unstructured dataset into ChatGPT and get good results. Much like training a team of interns, you need to give the AI agent team careful instruction about how to interpret the data it’s being fed. Even the differences between a New Business and an Upsell deal are enough to require additional guidance.
A deal story is also notably different from unbounded “chat with your data” AI experiences, which we think are still universally terrible. Even though this is a common feature request, we see people try them once, get underwhelming (or even hallucinated) results, and never try again. This won’t be the case forever — between technology improvements and end users becoming more familiar with how to interact with AI — but in the meantime, “working together with AI to create a shared asset” (such as one of these deal stories, or to configure a dashboard or add a column to an existing report) is a better paradigm.
The capabilities of AI are advancing rapidly, which means today is the worst these analyses will ever be. But even far more advanced LLMs won’t be the panacea, especially for any kind of quantitative analysis. For these use cases, we still need structured, clean data.
The road to true next-generation attribution involves next-generation data
We believe that the only true solution to the B2B attribution problem is through solving the underlying data problem first. If the dataset you start from is wrong — either because it’s missing records or contains duplicates — it doesn’t matter what quantitative attribution model you put it through. The results will be bad.
The traditional systems of record (like Salesforce or Marketo) aren’t trustworthy.
All B2B attribution models today are descended from an archaic, legacy data foundation based on lead funnels and designed to work primarily on single-buyer deals. It’s the structure that we all know so well from Salesforce: pre-defined objects like accounts/opportunities/leads/contacts/campaigns, and the static relationships between them:
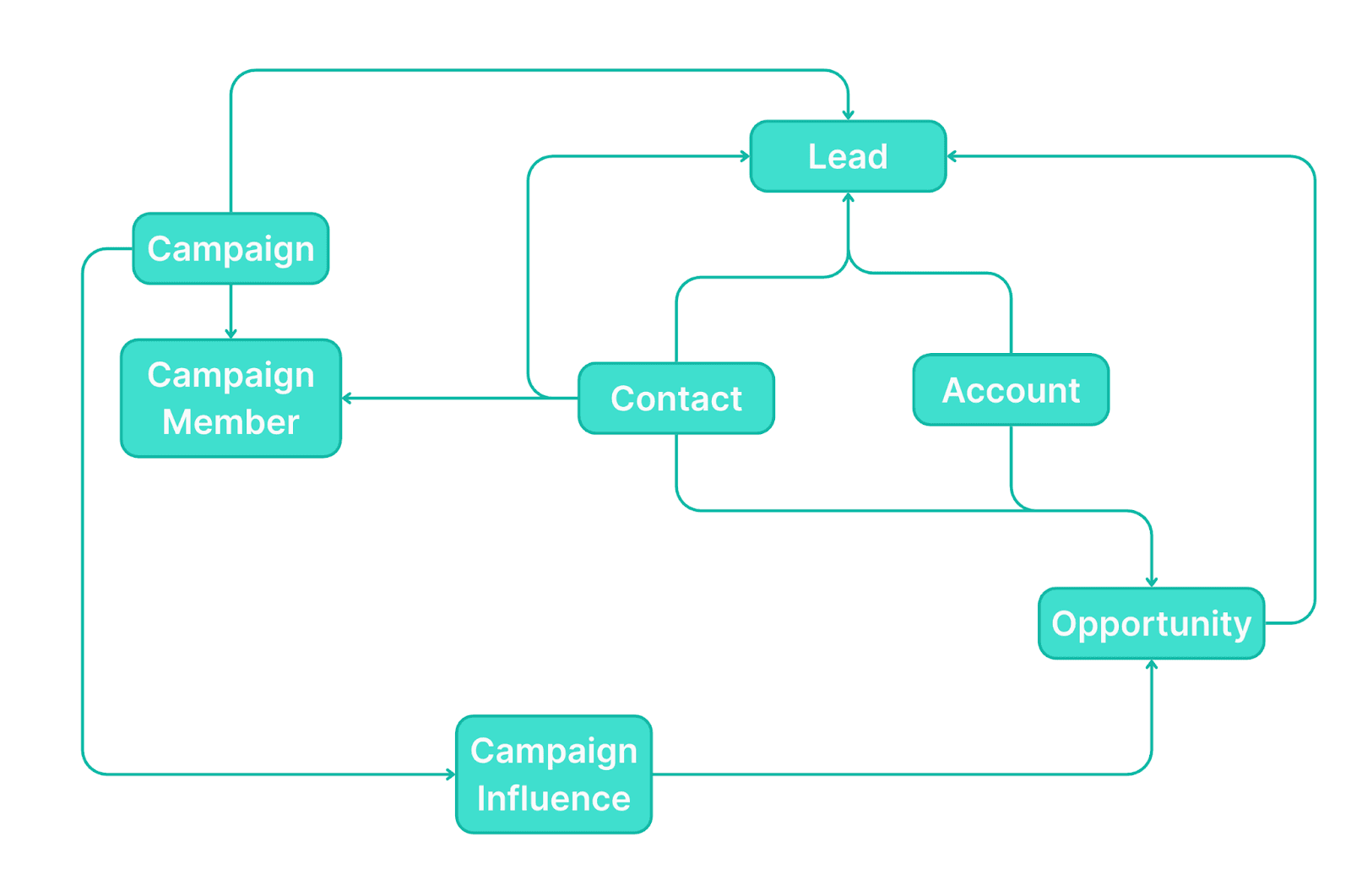
This foundation has served us all well enough in the past, and the problem is not with what it captures, but with what it misses. The language of Salesforce simply doesn’t have grammar to handle all the embedded signals when a prospect sends you an email like this:

We know this email is almost too good to be true (lightning does strike sometimes!), but the key insight for attribution purposes is that this prospect is a returning customer. Now, imagine this email arrives right after a website session with a chatbot conversation:

Any attribution tool based on a traditional data model is going to get this wrong every time — in fact, it will probably send you down the road towards a strategic faceplant, because it’ll tell you that “good deals come from chatbots” when the truth is that the chatbot simply happened to be the most convenient button on the website for someone with pre-existing high intent.
A next-generation attribution model needs access to these hidden and buried signals.
How do you build a next-generation data foundation?
At Upside, our mission to build a better data foundation for next-gen attribution has forced us to make a big mindshift: the traditional systems of record (like Salesforce or Marketo) aren’t trustworthy. The data inside them is an extremely useful source of fuel, but not a source of truth.
For example:
- An AE might not add someone to Salesforce as a contact, but will add them to a calendar meeting. They might not log the coffee meeting or quick call, but will send a follow up email after.
- Marketing teams might not enter the exact dates for a VIP event, but will add people to campaigns and send invitations with the right date inside.
The goal is not to “clean up” the data coming from these sources — it’s much more transformational: to reconstitute the most-likely-correct version of what happened based on all the signals available.
We’ve been working on this for over a year and still don’t have all the answers, but we’re starting to see breakthrough improvements. Here’s the outline of how we are approaching the problem now:
Step 1: Ingest raw data, and create a dense graph of signals
Signals exist everywhere. If you’re capturing data, it contains signals that could be useful. Systems like Salesforce and Marketo or HubSpot are the places to start, but more is better.
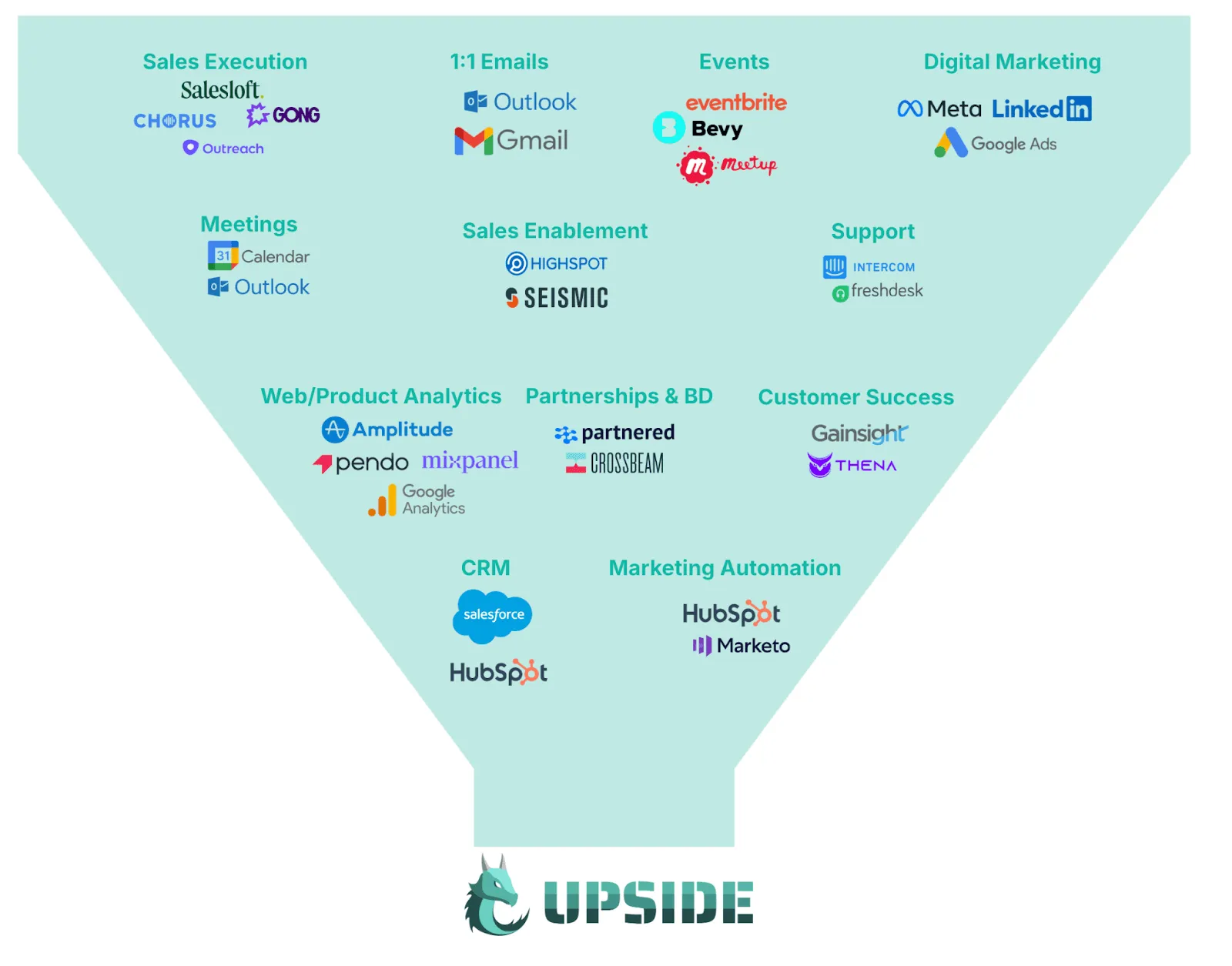
ETL tools like Fivetran make this process much easier, because they have prebuilt integrations with most main sources. In practice, we’ve found that we can usually get pretty far with just Salesforce and Marketo/HubSpot because mature go-to-market-organizations tend to already treat Salesforce as their de facto data warehouse: the raw data ends up dumped in there somewhere, even if it isn’t addressable for any sort of meaningful analysis.
We’re extracting signals not only about the touchpoint itself, but also about all of the “phantom touchpoints” referenced within it (for example, the casual mention in an email of “coffee yesterday”).
At this stage in the process, the goal is not to create the final dataset for reporting because there will be a lot of overlap. We’re extracting signals not only about the record itself, but also about all of the “shadow records” referenced within it (for example, the casual mention in an email of “coffee yesterday” — we call these “phantom touchpoints”). Conceptually, it’s a little like letting bread dough rise, and the output is going to be a giant, extremely messy graph with literally billions of individual “nodes”:
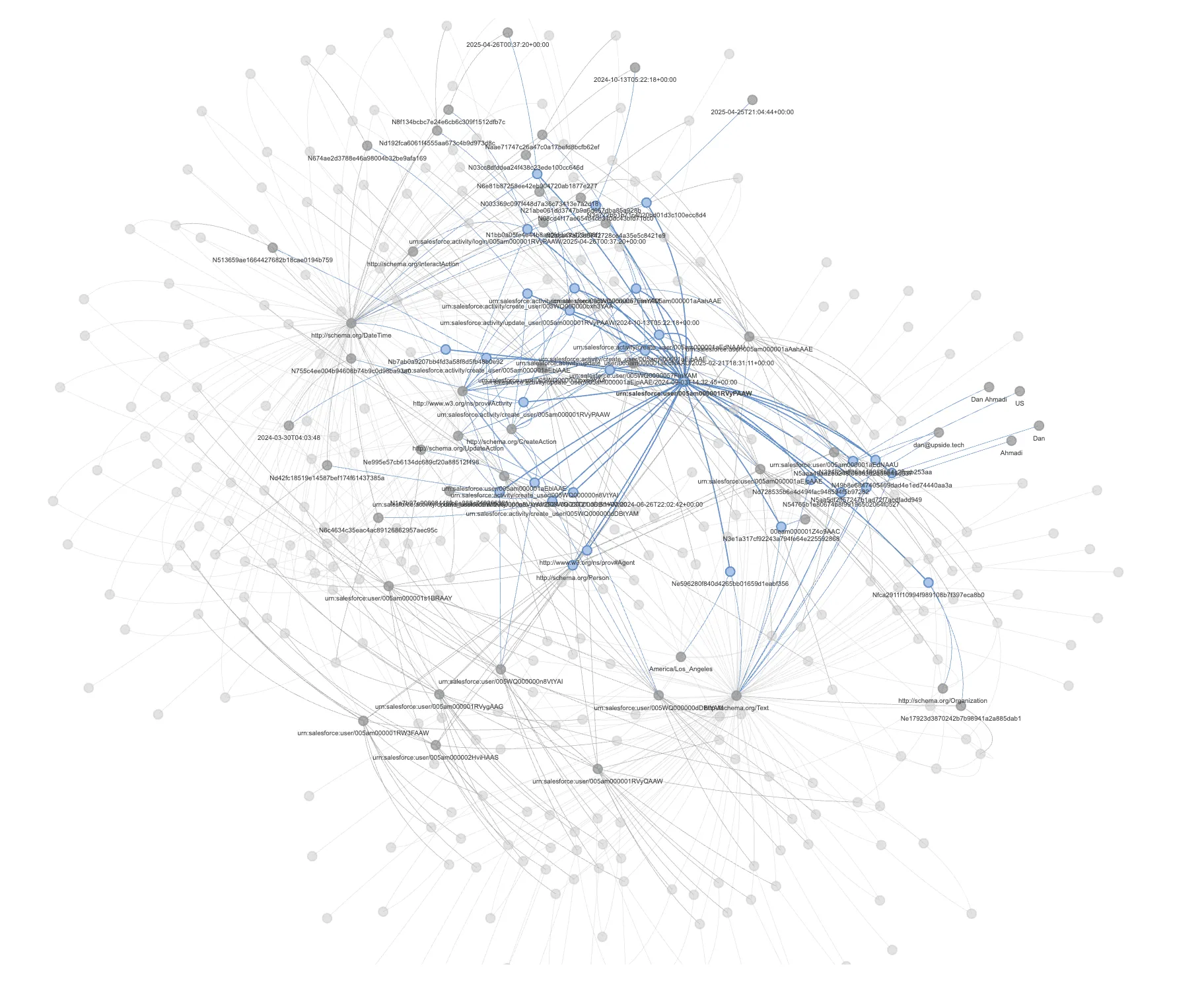
This is ultimately a structured data problem, not dissimilar to what a search engine like Google does when crawling the web. We’ve been bootstrapping our work with industry standards like Schema.org and PROV-O, which provide extensive references for how to map real-world activities into structured data. We’ve also been testing ways to use AI to speed up the mapping of data sources into these schemas.
Step 2: Use entity resolution to synthesize a canonical version of what happened
Once you have a giant, semi-structured soup of overlapping signals, it’s time to distill back into a set of canonical records. Entity resolution is a well-established discipline, and we’ve been researching a variety of techniques to understand which ones give the best results with go-to-market data.
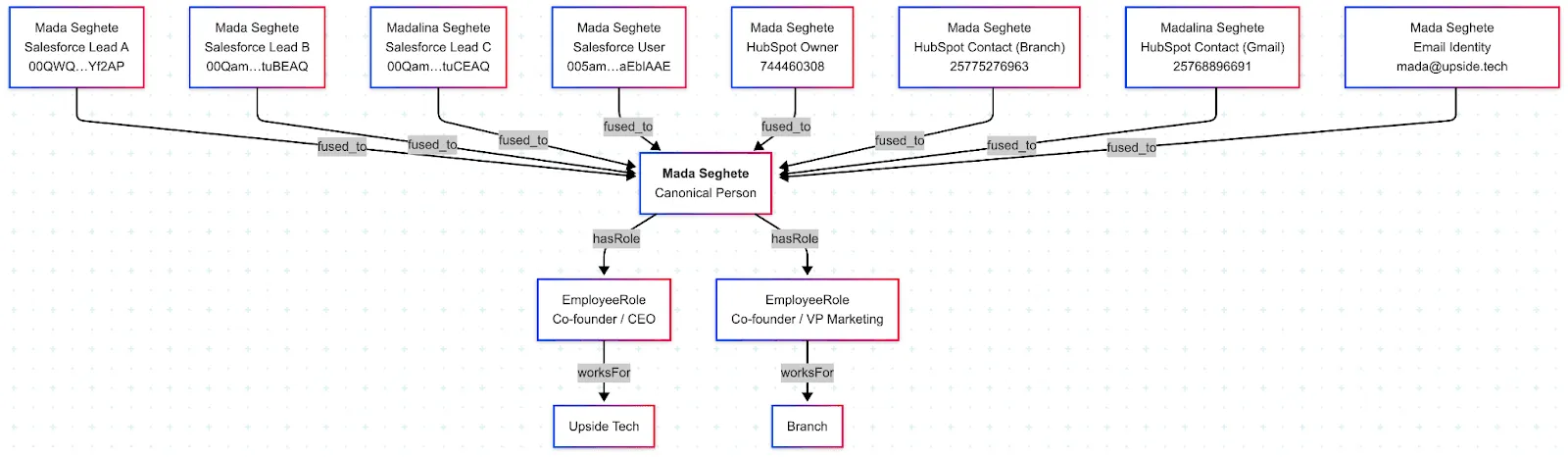
The goal is to create a comprehensive dataset, equivalent to traditional objects like people/accounts/campaigns/touchpoints, but with far fewer missing pieces. In Genesis, our data foundation, we sometimes refer to this as “the blood-brain barrier.” Downstream analytics systems (such as the attribution model) no longer need to deal with the chaos of data in source systems like Salesforce or Marketo because they’re receiving a clean feed of deduplicated, healed data. In the example above, Genesis has correctly identified that the “Mada Seghete” who founded Branch is the same “Mada Seghete” who founded Upside, even though there is no direct link between these records.
What you can do with this data
Comprehensive “mission control” for your account stories
People instinctively distrust black boxes, and want the option to drill into the raw data behind any roll-up number or analysis to confirm it is believable.
One of the first customer-accessible tools we built was an “account story viewer” because it was something we needed ourselves on a daily basis (jumping between Salesforce, Marketo, Gong, and half a dozen other tools to debug an account story gets old, fast).
We’ve talked to a number of teams who built a similar tool in-house (often in a platform like Looker), because nothing comprehensive exists on the market for it today. But these still suffer from the flaws of messy source data, and also inherit all the typical challenges of in-house tooling, including unpleasant UX and frustrating load times with larger datasets.
Some of the key features we’ve needed for this include:
A “minimap”
A “minimap” (like in a video game) that gives a clear visual representation of when interesting things are happening in the account. This includes when opportunities were open and progressing, when new people showed up for the first time, and when the account had velocity (calculated by showing customer engagements against the “bids” coming from teams like sales and marketing). The minimap helps to-go-market teams see where interesting things were happening, and jump straight to that moment for further investigation.

A chronological log of touchpoints
A chronological log of touchpoints showing who engaged, in which channel, with which go-to-market team, and so on. This supports multiple filter modes, so you can drill in and understand the impact of every campaign or person on a deal. Once you see what happened, you can re-engineer the most effective thing to do on similar accounts going forward.
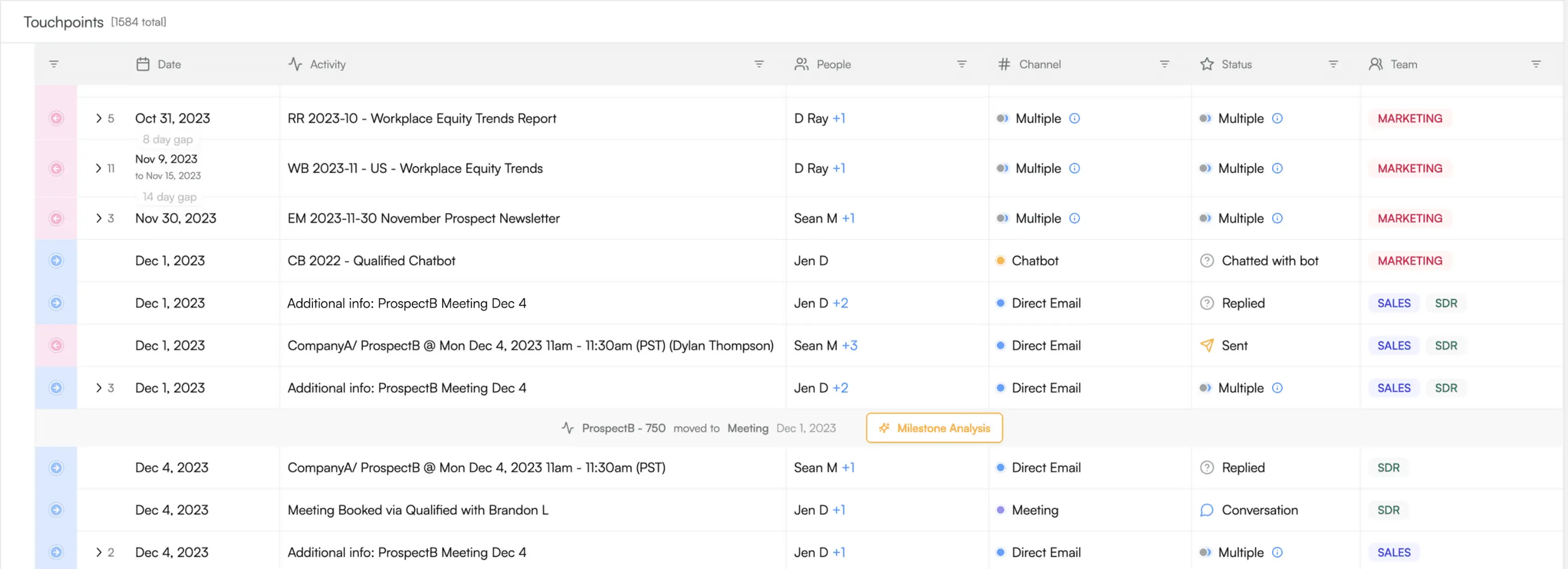
Summaries of “milestone moments”
Summaries of “milestone moments” to make sure that people don’t miss the forest for the trees about what drove progress in the account. These summaries cite their sources, so that it’s easy to jump back into the full deal story for follow-up investigation.
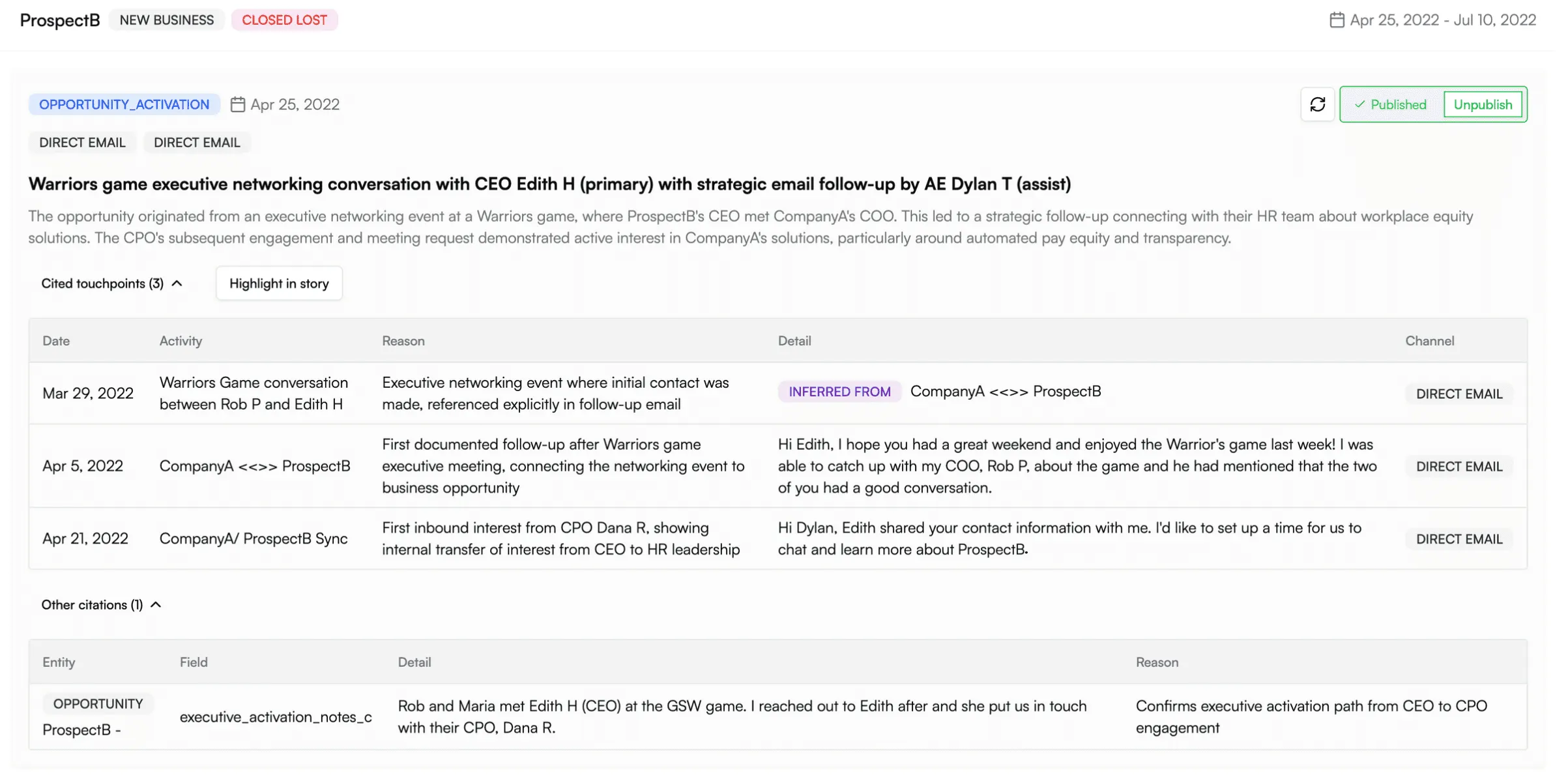
Deep touchpoint forensics
Deep touchpoint forensics so that people can dig into all the details in one place without having to jump between different platforms. In our platform, this already includes information like sales email content and web session details, and we are expanding it to cover additional data types like call transcripts, marketing email content, and chatbot conversations. This dramatically simplifies the task of finding all the hidden handoffs that happen in a typical enterprise deal cycle.
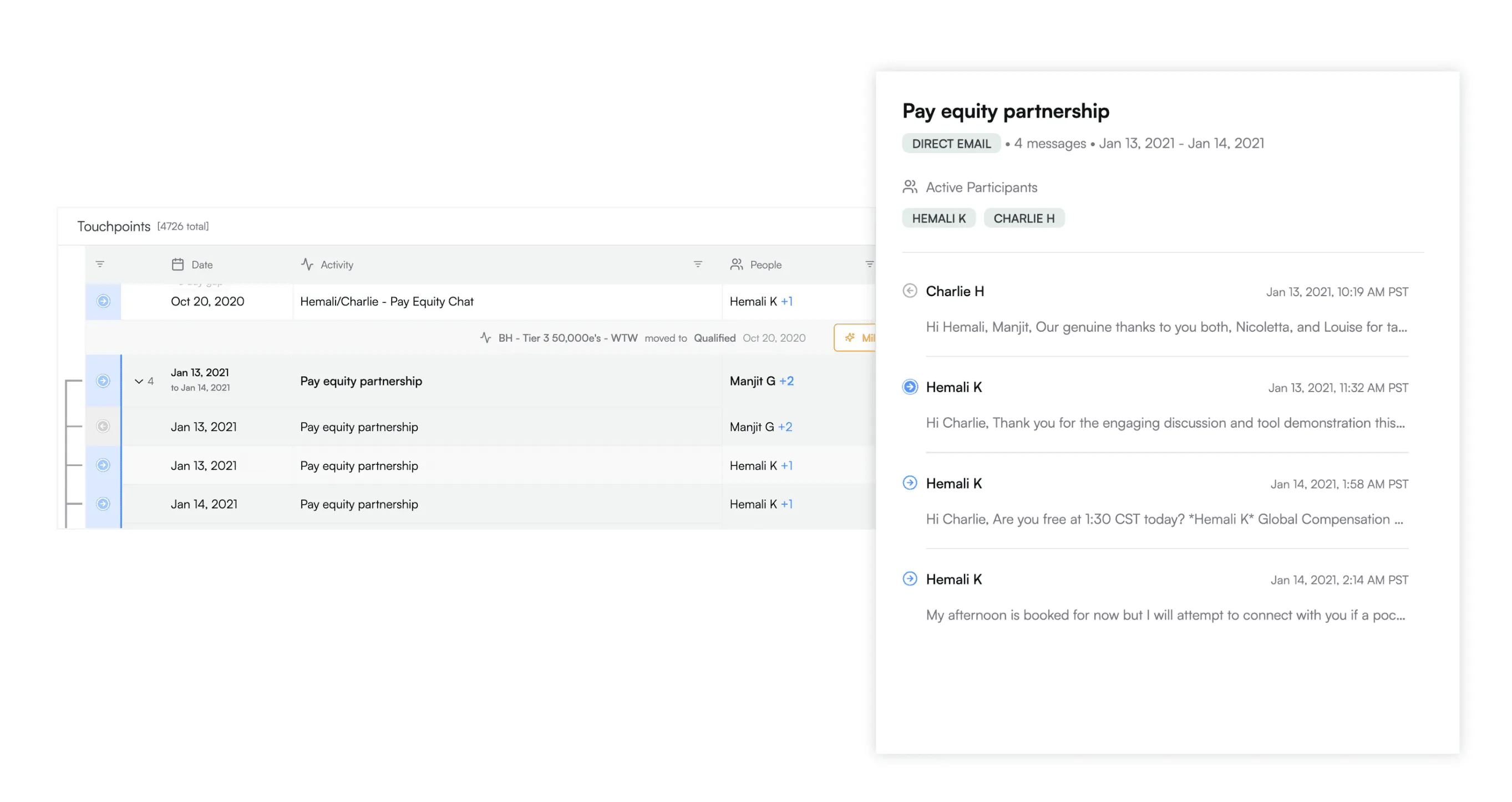
Campaign and channel scorecard reporting
Every B2B go-to-market organization needs to report on KPIs for their investments. This is often an exercise in vanity metrics, because real data is so painfully tedious to pull. But with a comprehensive account story for every prospect, it suddenly becomes much easier to generate meaningful insights for each campaign. For example, the number of opportunities activated or progressed after an interaction with that campaign, combined with the ability to drill down to the deal story and check how that activation happened.
With this level of insight consistently available to everyone on the team, it’s much easier to make good decisions without waiting for a QBR or an overloaded BI analyst to pull one-off data reports.

Customer acquisition cost and deal ROI
Once you have a comprehensive audit of the touchpoints involved in closing a deal, you can attach costs for all of them and estimate how expensive it was to land each customer. These insights help you make decisions about which industries, geographies, and customer tiers are worth pursuing. We’ve already started running proof-of-concept experiments for this, and expect to start working on it in the near future.
Weighted attribution
We know that assigning credit for pipeline or revenue dollars to specific activities is still an attractive goal for many teams because it enables zero-sum reporting where everything adds up. We’ve continued to monitor the improvement of our original impact-weighted model as the underlying data quality improves, and we’re also researching other approaches to weighting schemas that were not possible within the limitations of a traditional data foundation. One promising possibility includes a predictive model that allocates credit to each touchpoint based on how much it increases the likelihood of a deal closing.
Next-best-action predictions
This is one of our most common customer requests: after you know what has helped close deals in the past, you can equip every person on the team with insight about the best step to take next for each deal that is open today. This is also one of the most highly-leveraged modeling exercises, which means it’s extremely important to be confident in the stability and quality of data at lower levels first.
Takeaway: B2B attribution CAN be great, and it’s worth the effort!
A year and a half into this journey, we’re more convinced than ever that game-changing measurement is possible, and that using reliable data to get the full picture of your growth engine is one of the best things you can do to scale your business.
We have helped our design partners uncover what drove their largest deals, given insights to C-level execs to inform shifts in their inbound vs. outbound spend, and shown teams which signals truly mean a deal has a high likelihood to close.
We also have a far clearer picture of what it will take to make meaningful recommendations about what to do next, taking into consideration contributions from the whole go-to-market organization, and why traditional approaches to attribution and prediction are continually doomed to failure.
If you’ve been trying to solve this problem for years, somehow never managed to make progress, and are now looking for a more rigorous approach to your GTM insights, we would love to hear from you. Please reach out to us and we’ll be in touch!